26th October
"Machine Learning for exploration: review of current and future tools"
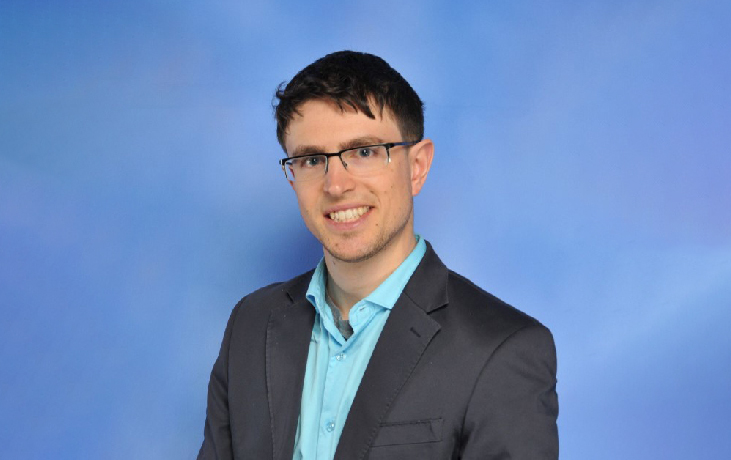
Dr. Antoine Caté, Senior Consultant in Structural Geology at SRK Toronto, will lecture on this topic at the next edition of the International Congress of Prospectors and Explorers - proExplo 2021. Below you will find an interview with Dr. Caté.
How did you become an expert on “machine learning” applied to mineral exploration?
I was first introduced to machine learning during my graduate studies in Quebec. The project required to integrate a large multi-element lithogeochemical dataset over a deposit. We realized we needed machine learning to answer the problem. I then continued to learn through both academic and industry-related projects. Machine learning was just starting to become a subject of interest at the time and I had the chance to be at the right place at the right time.
Can you please define for non-experts the meaning and relationship between “big data”, “machine learning”, “neural networks” and “artificial intelligence”?
The term “big data” references any dataset that is too large to process, analyze and visualize with standard software. A dataset can be large due to its volume (number of data points), its variety (the complexity of each data point) and velocity (the speed at which the data is generated). The vast majority of datasets in exploration geology cannot be classified as big data.
The term “machine learning” references to all computer algorithms that make predictions based on example data. Example data is provided to the algorithm, the algorithm learns automatically and with minimal human input the patterns in the data, and it can make predictions based on this learning. For example, an algorithm can be provided thousands of images of rhyolite and basalt. The algorithm will learn the difference between the two and will be able to predict if a new image is a rhyolite or a basalt.
A neural network is a network of neurons or nodes connected between each other by weights. A weight represents the strength of the connection between two neurons. Neural networks architecture is inspired by biological neurons in the human brain. The neurons are connected in a given order with the value of a neuron being determined by the value of all the input neurons multiplied by the connecting weights. As a simple theoretical example, input values such as assay data are provided to a network. The assay data is multiplied by the connecting weights to calculate the output neuron. If the output neuron has a low calculated value, the sample is barren. If the output neuron has a high value, the sample is mineralized. Complex neural networks with millions of interconnected neurons can be used for complex tasks such as automated core logging using core images.
The field of artificial intelligence focuses on the research regarding computers ability to think and reason like a human. However, in a more down-to-earth explanation, artificial intelligence refers to all software or algorithms that mimic human cognitive functions. This definition encompasses a large spectrum of artificial intelligences. For example, an Excel cell that calculates 1+1=2 is an artificial intelligence. A self-driving car is also an artificial intelligence.
Can you tell us about a success case of discovery driven by the use machine learning?
Machine learning cannot be independently responsible for having any discoveries whatsoever. Machine learning has been used with other tools to make discoveries in mineral exploration. There are several examples of companies such as Goldspot Discoveries or Orefox which have generated targets using machine learning and have validated them in the field. However, machine learning is just one of the combined array of tools that lead to the discovery. Good data collected in the field or through remote-sensing, sound exploration model and skilled field geologists validating the targets are all necessary parts of the discovery process. Machine learning is just a powerful tool that helps mineral exploration, similarly to geological mapping and structural analysis.
Do you think this pandemic world we all now live on may accelerate even more the use of computer-based processes in mineral exploration?
I totally agree with this statement. The pandemic was a good opportunity to take some time to look at our data and to extract value from it. We also had to find ways to continue exploration without being able to access the field. And this generally goes through a computer. As an example, we had to rely on logging core using core images during the pandemic. It was also an opportunity to develop new tools. We had to build an algorithm that automatically maps all the veins from drill core images of a deposit for a client as manual relogging would have been too costly and time-consuming. I think the pandemic was a good opportunity to optimize our work and improve the exploration process. Despite these opportunities, the pandemic has reminded us how valuable field work and site visits are.